Machine Learning
Machine Learning
Our IP Machine Learning bundle brings together leading algorithms for performing classification and curve prediction based tasks.
Carry out data repair, prediction of missing curves and key reservoir properties from minimal source data using our Neural Networks, Fuzzy Logic and Multiple Linear Regression modules.
If you are looking to classify your well log data into distinct facies or rock types, then our Cluster Analysis and Self-Organizing Maps modules have you covered.
Unique to IP is our Domain Transfer Analysis (DTA) module which provides a mathematical advantage over other methods for prediction of missing and incomplete data. Using n-dimensional Partial Differential Equations, non-linear relationships can be revealed that other tools miss - giving its results that vital extra edge of accuracy.
A common interface between the modules allows fast and efficient model comparison.
Explore the modules
included in this toolkit
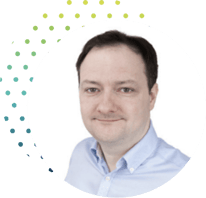
Andy Mcdonald
IP Product Champion
“Adoption of machine learning and artificial intelligence techniques are becoming increasingly popular within the geoscience domain. It can bring many benefits that improve petrophysical workflows, including automation, missing data prediction and lithology classification.”
Curve Prediction
Applicable at any stage of a well's life, Curve Prediction enables advanced interpretations from minimal source information. Employing several statistical methods, Curve Prediction's tools help you to generate new curves from offset wells, repair existing data, or even make continuous curves from discrete data.
- Predict key reservoir properties including porosity, permeability and saturation from log curves and core data
- Infer missing data or repair incomplete well data from bad hole intervals
Pictured: Crossplot your prediction results against actual measurements.
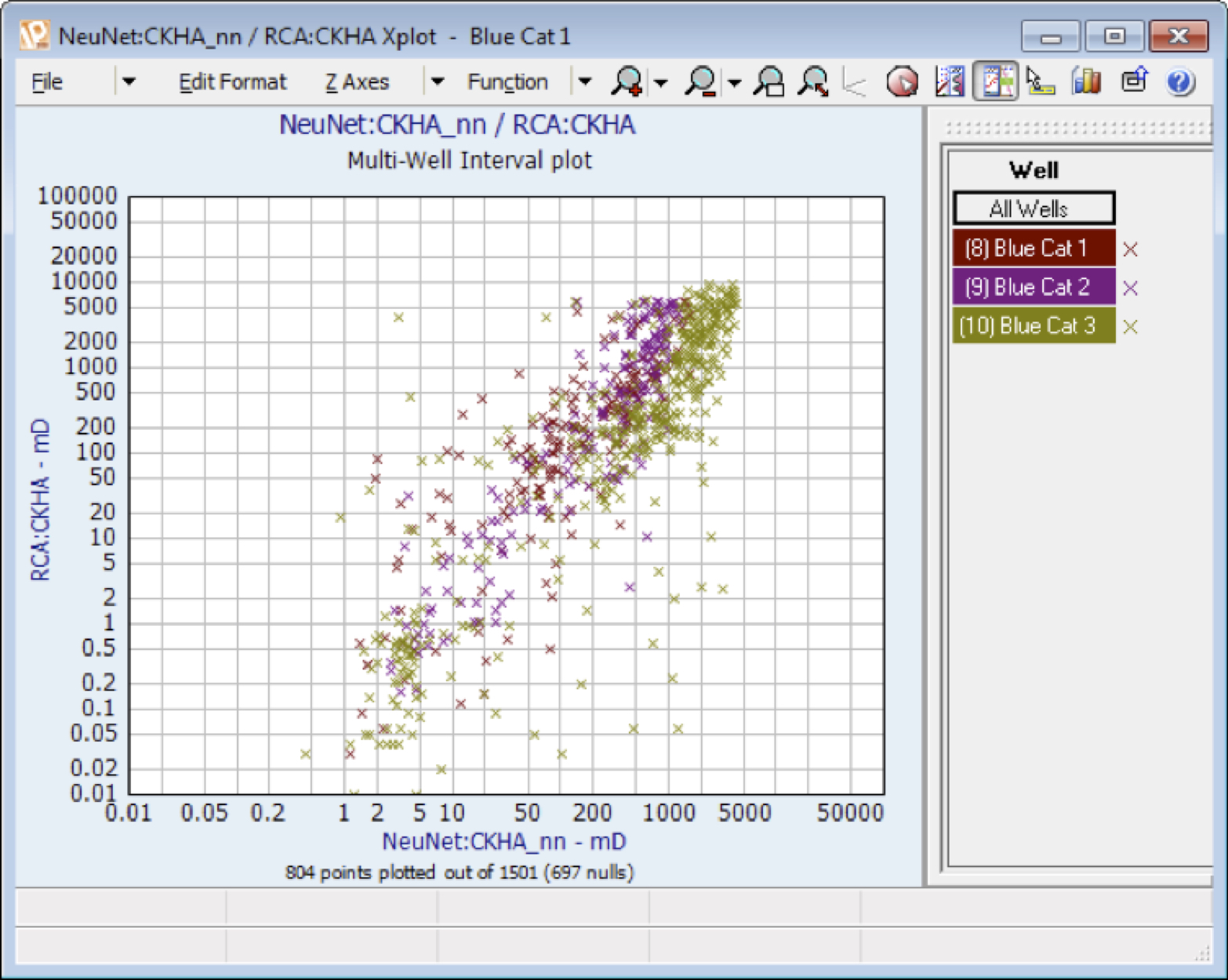
Domain Transfer Analysis
Domain Transfer Analysis (DTA) is our premier solution for porosity, permeability and saturation prediction. Create multivariate and non-linear predictions based on real data from the current well, nearby wells or their sidetracks – you can even include previously unused information from normal deterministic analyses.
DTA's key advantage is mathematical. It employs n-dimensional Partial Differential Equations to reveal non-linear relationships that other tools miss – giving its results that vital extra edge of accuracy.
Pictured: Setup and Zone Selection within the DTA module.
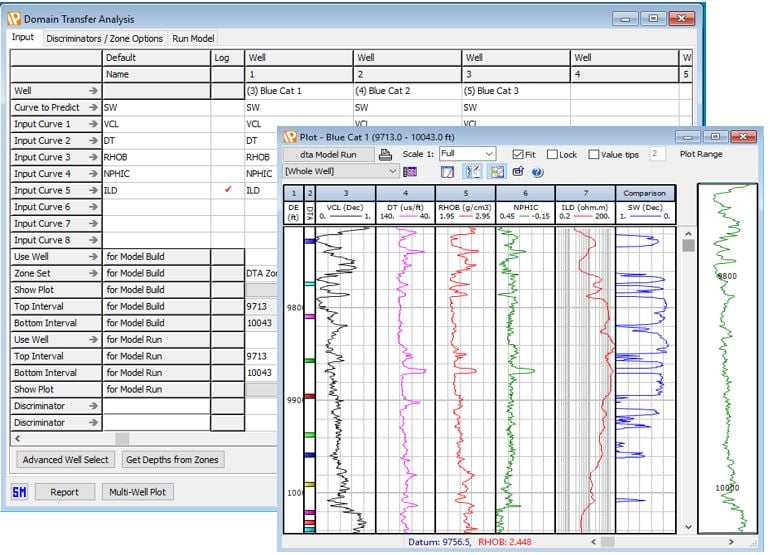
Rock Typing
Rapid and easy to learn, Rock Typing employs powerful algorithms to build repeatable and accurate facies classifications. With both Cluster and Self-Organising Map tools, you can analyse core or raw log data to cluster, zone and focus on the best approach to lithology, porosity and saturation.
- Build and apply a facies model to any number of wells
- Cross-plots and starplots graphically show how key input parameters affect facies selection
- Compare any Rock Typing models in Contingency Table, including core data and manual picks
Pictured: Visualise the results of Self Organising Maps in 3D or 2D.
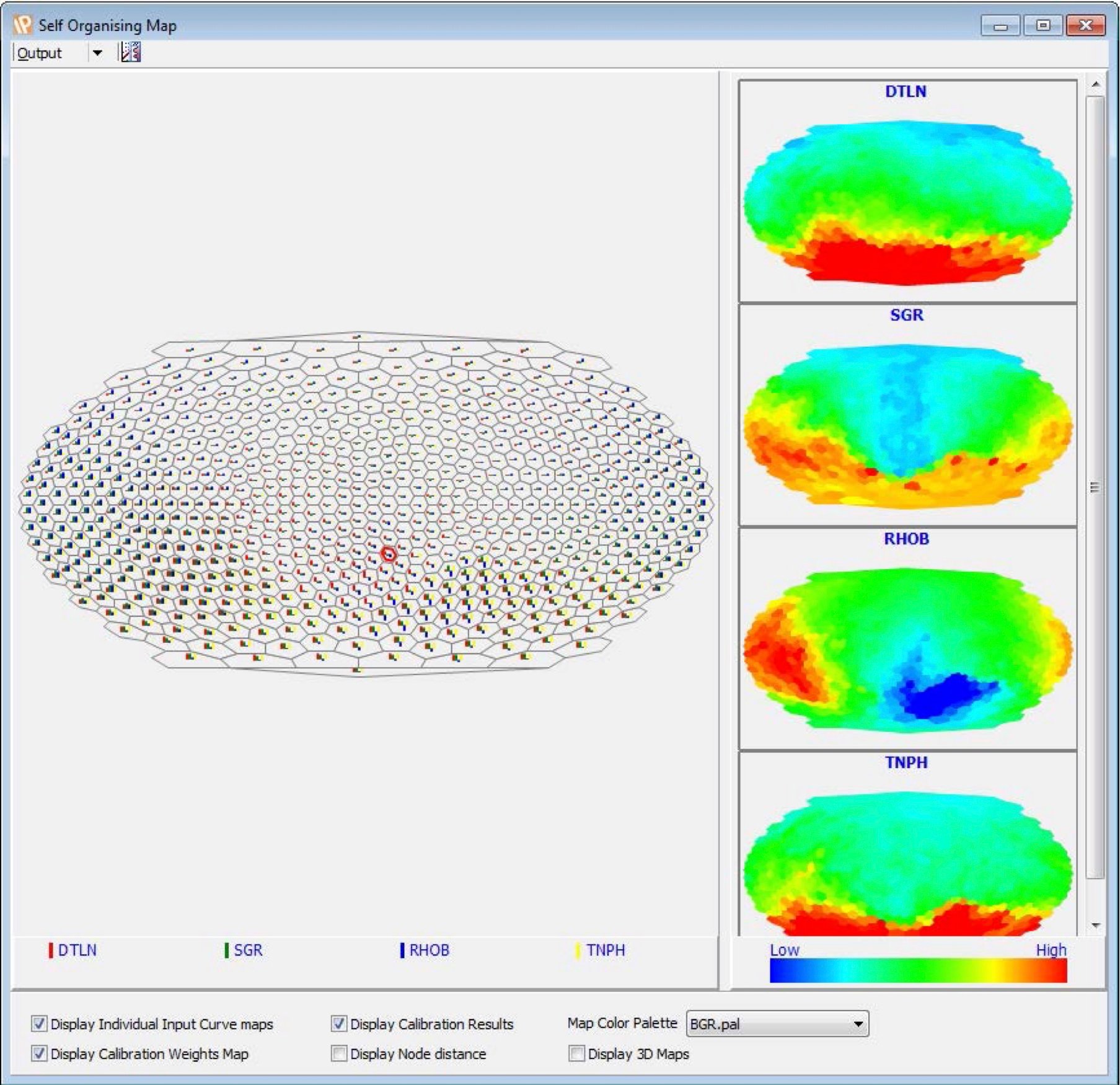
Explore IP
From porosity and pore pressure to reservoir productivity, IP gives you an incredibly capable and customizable solution to share and interpret well logs and many other vital data types.
Toolkits
IP Foundation
Contains all the tools and modules required for the complete management of subsurface log data including loading, log plots, x-plots, histograms, editing, filtering, depth shifting, exporting etc. Carry out the full, deterministic, petrophysical workflow – Includes Clay Volume, Porosity/Water Saturation, Cut-Off and Summations modules plus NMR Interpretation. Also includes Monte Carlo Analysis for uncertainty analysis, Chrono Log, IP Power Tools and the ability to create you own IP applications.
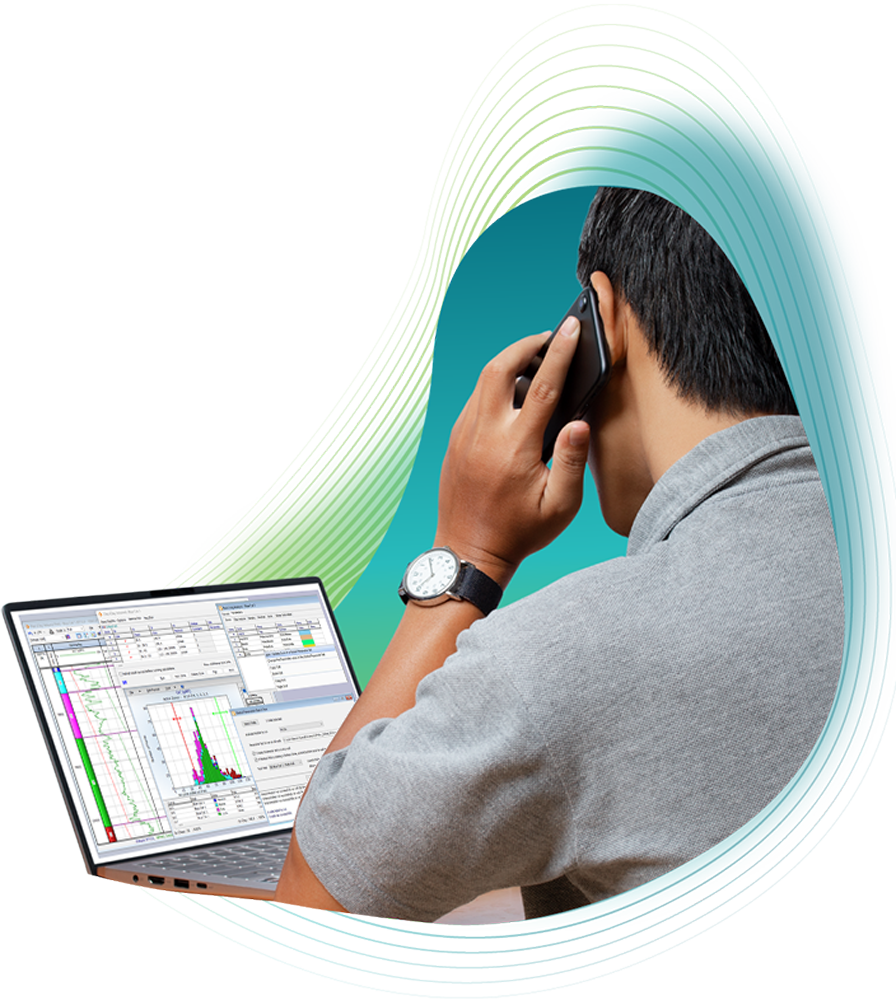
Advanced Formation Evaluation
Carry out complex interpretations with Mineral Solver, and characterize your reservoir with Saturation Height Modeling, Hydraulic Flow Units, and Formation Testing modules.
Learn More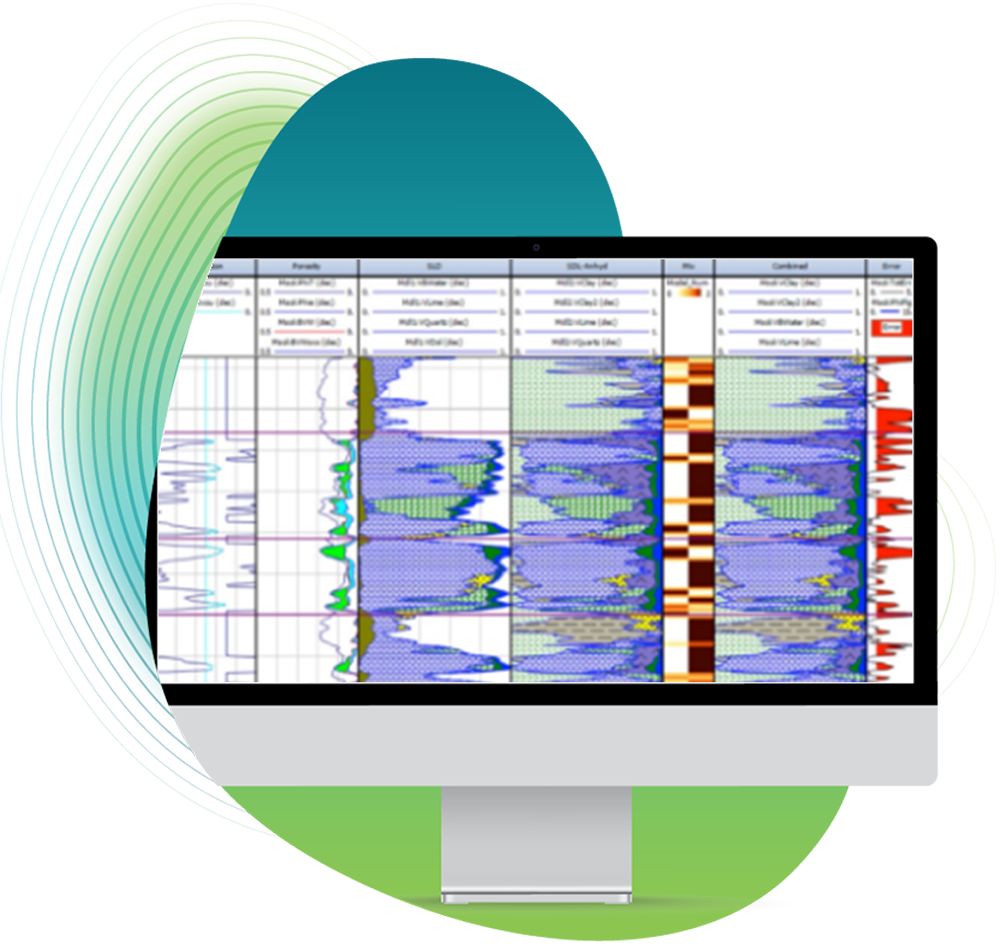
Borehole Imaging
Perform enhancements and corrections to Image Log data, utilize the vast amount of display and interpretation options specifically tailored for all the main image log tool types on the market.
Learn More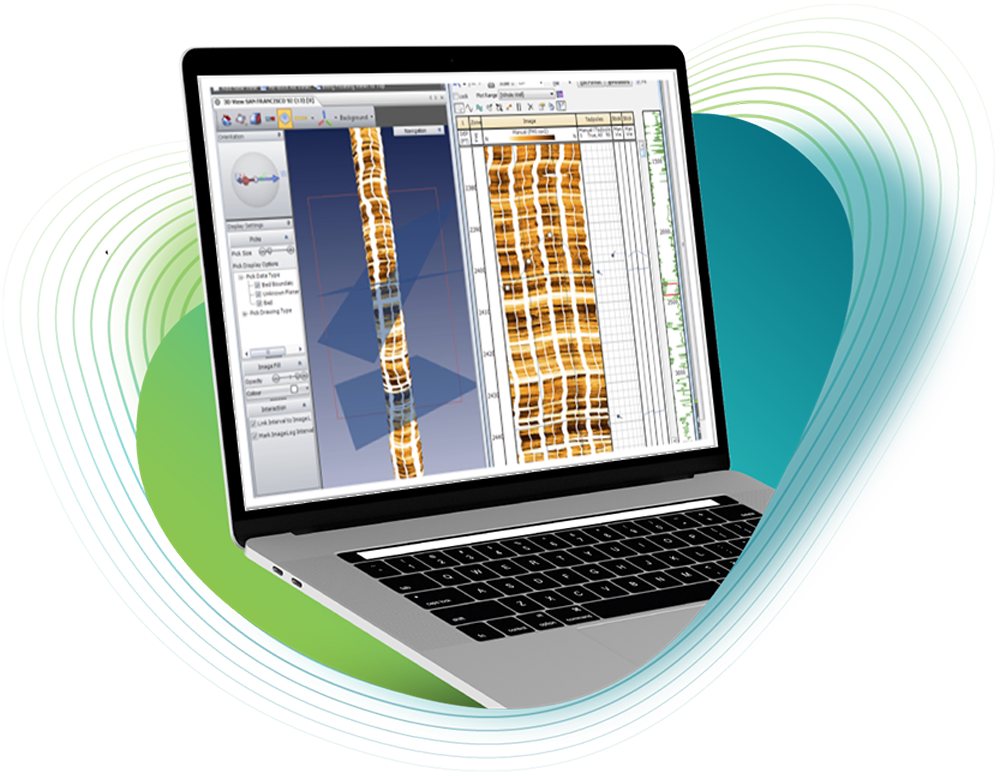
Cased Hole
Assess the downhole integrity of your wells with the casing and cement evaluation modules, while also monitoring reservoir fluid saturations and the production performance of each well.
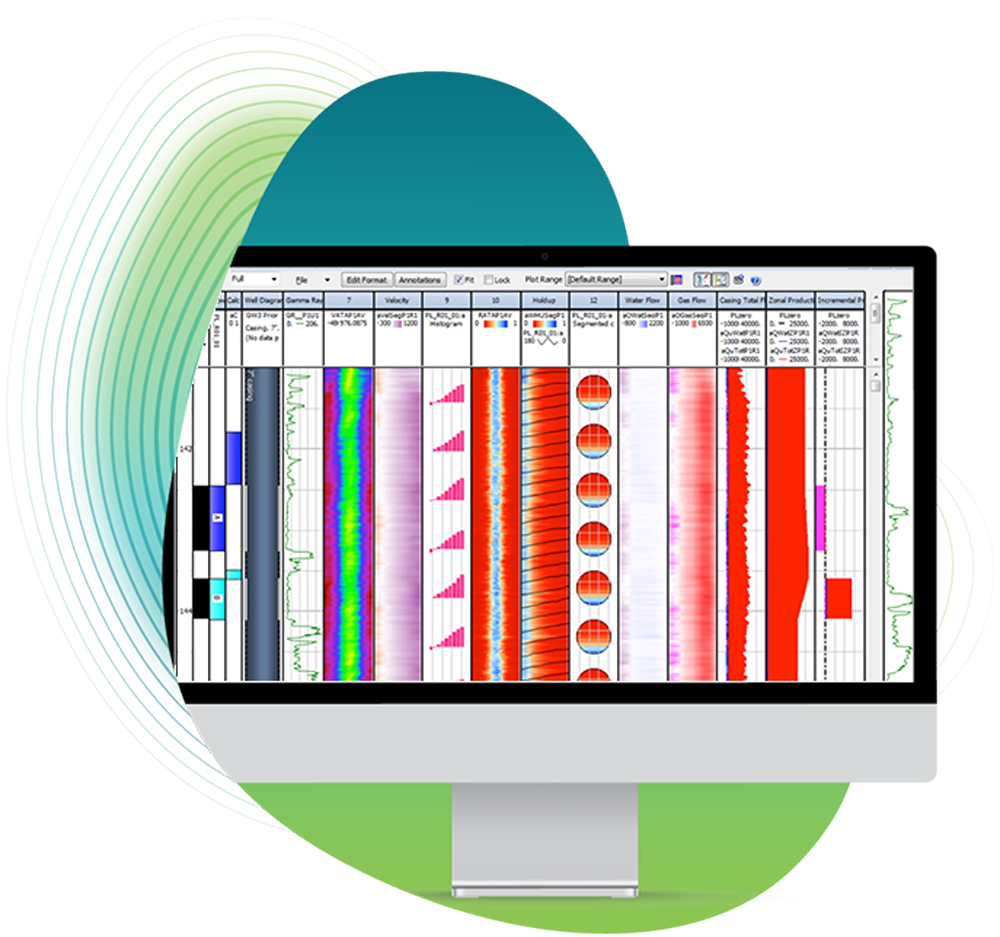
Geomechanics
Assure your well’s stability and maximize production lifetime using our suite of Geomechanics modules. Calculate pore pressures, determine wellbore stability and calculate risk of sand production.
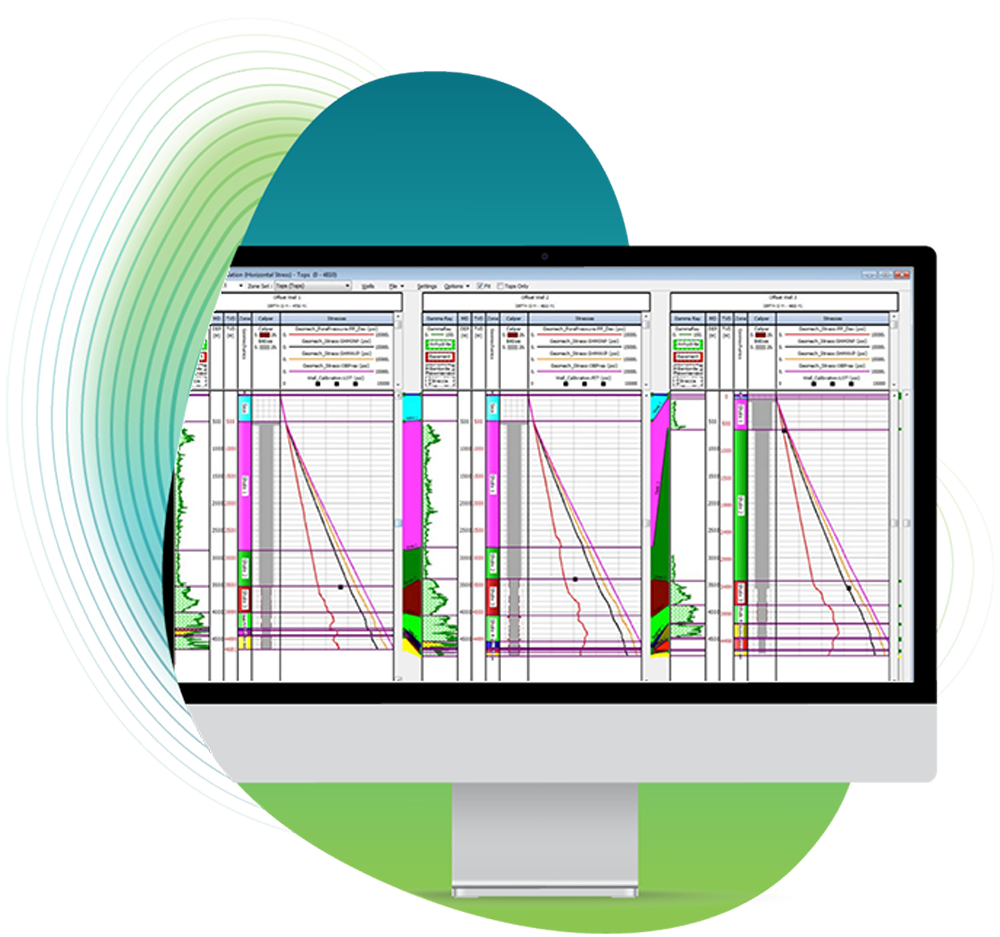
Geophysics
Bring your Petrophysics and Geophysics teams together to calculate the downhole acoustic properties of the formation, carry out fluid substitution analysis and create synthetic seismograms.
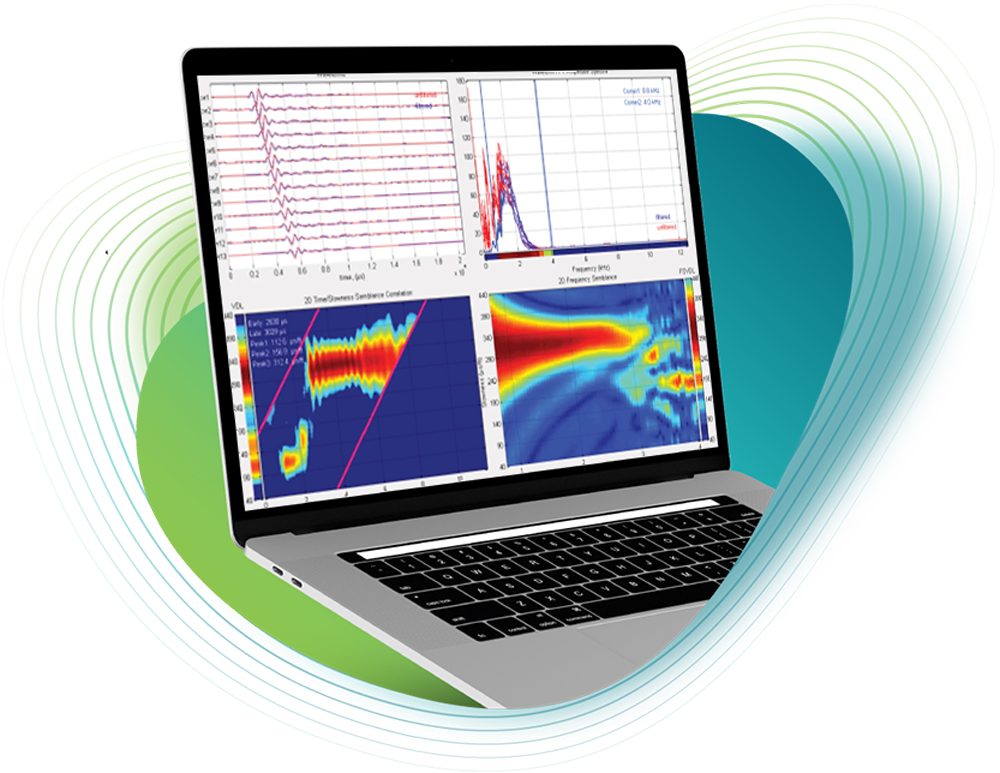
Machine Learning
Carry out data repair, prediction of missing curves, or key reservoir properties using minimal source data. Classify reservoir rock types using Self Organizing Maps and Cluster Analysis.
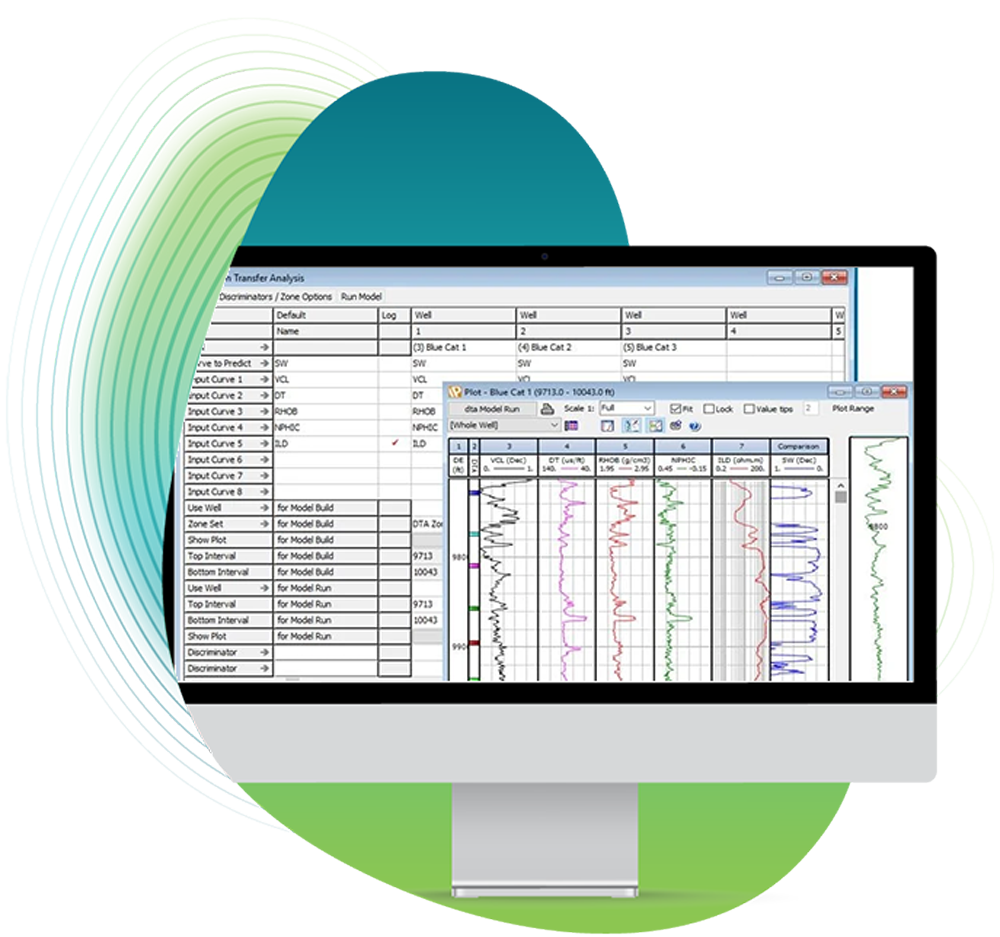
North Sea Database
Our database is designed to streamline exploration efforts across the Norwegian North Sea. Providing DISKOS members this powerful tool will save you time and money, offering first-hand verified data that ensures accuracy and reliability.